A Technical Explainer on Apple’s Concerning Privacy Changes
Brief
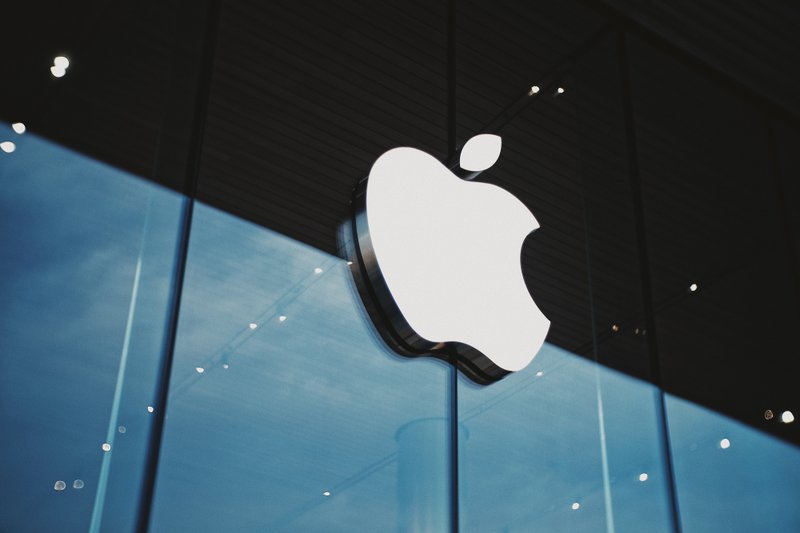
Sukrita Rungroj/shutterstock.com
Aug. 30, 2021
On August 5th Apple released new plans for how it handles users’ data on the iPhone and iPad platform, some of which have significant and very concerning implications for user privacy. In a new page on its website, entitled Child Safety, Apple announced updates that it is making to its operating systems in an attempt to address the problem of Child Sexual Abuse Material (CSAM). While the changes were all presented at once and described on the same webpage, there are actually three distinct changes that affect different categories of people and different Apple products. A deeper understanding of how the tech works is essential to understanding why Apple’s safety changes create a framework for potential mass digital surveillance around the globe. While we support the important goal of promoting child safety, we have concerns about the broader impacts of Apple’s strategy. OTI has joined over 90 other civil society organizations urging Apple to abandon these proposed changes due to threats to privacy, security, and free speech for everyone who uses an Apple product. This piece lays out the major technical impacts of the changes, including Detecting and Flagging Images in Messages, Scanning Photos Stored in iCloud, and Changes to Siri and Search Results.
Editorial disclosure: This brief discusses policies by Apple, Dropbox, Google, and Microsoft, all of which are funders of work at New America but did not contribute funds directly to the research or writing of this piece. View our full list of donors at www.newamerica.org/our-funding.
Detecting and Flagging Images in Messages
The first update Apple announced is the addition of features to the Messages app that are designed to prevent children from sending or receiving what it describes as “sexually explicit material.” This change applies to messages sent over Messages to other Apple users, and those sent through SMS from Apple users to non-Apple users, both of which use the Messages app. In order to enable this feature, the device must be enrolled in a family plan, the owner of the plan (an adult who administers “Family Sharing” on an iCloud account) has to designate the device as belonging to a child under 18, and the owner of the plan has to turn on the protection for the device.
Once this feature is enabled, a machine learning algorithm begins running on the device that evaluates images being sent or received through the Messages app to detect what Apple describes as “sexually explicit material.” Apple claims that the algorithm is not “just a nudity filter” but that “a nudity filter is a fair description.” When Messages determines an image a child user is receiving does fall into the “sexually explicit” category, it is blurred out and the user is informed that the image may be sensitive. The user can choose to reveal the blurred photo regardless, and is then presented with a screen giving information about sensitive photos and asking if they really want to continue. Likewise, when the algorithm detects sexually explicit material in an image the child is sending to another user, the sender is also warned and provided similar educational information before the photo is sent.
In addition, if the user of the device has been designated by the owner of the family plan as being under 13, the device will notify the owner of the plan if the user chooses to still send or view the photo despite “sexually explicit” warnings. That notification will not contain the message or image itself. While Apple will not receive notifications of those choosing to bypass “sexually explicit” warnings, it is not clear whether Apple will be able to access any records of these notifications and user choices, and if so, for how long. Even if Apple doesn’t currently log these notifications there is nothing in the design of the system that prevents it from doing so in the future.
There are a few important technical details to note. Importantly for privacy and security reasons, neither the message nor the image itself leave the user’s device. This means that the message and image remain in the control of the account user, rather than being shared with a third party for evaluation. The machine learning analysis is conducted completely on the device by software built into the iOS 15 operating system that runs on every Apple mobile product. In the event that a notification needs to be sent to the plan owner, that notification does not contain the relevant message or image.
This system nevertheless poses several concerns. First, there are many documented examples of machine learning algorithms used for image detection with significant false positive rates. Constructing a filter that reliably reports pornography while excluding images that are medical, fine art, or simply of people in bathing suits, has never been truly accomplished. Such nuance typically requires a human in the loop who can review and analyze for context, as OTI has recommended in cases where content moderation relies primarily on AI for initial sorting of content. These algorithms are also subject to intentional adversarial attacks, in which the algorithm can be shown a manufactured image that causes a false positive with a non-explicit image or even potentially a false negative with an explicit one.
This machine learning filter could also be used to identify other categories of images in the future, especially as governments around the world see the potential of such a feature. If Apple builds in the ability for specific “types” of content to be scanned for and filtered, then governments will likely want it to also identify “terrorist” or “extremist” content, certain types of hate speech and symbolism included in an image, and beyond. However, because governments around the world certainly don't agree on definitions for these categories, they could exploit this vagueness to carry out political targeting and other surveillance. And further, countries that outlaw political dissent may try to exploit this new feature given the opportunity to target vulnerable groups like activists, journalists, and political opponents. Even if the images being sent are not shared with Apple, receiving a notification and warning that an image may be illegal in a certain jurisdiction can have a chilling effect on free speech and communication.
Notably, Apple cannot verify whether members of the Family Sharing plan are children or other adults. The owner of the plan is likely to be whoever set up the main Apple account in the first place. That person can then designate any other device on the plan as a “child,” either under the age of 18 or under the age of 13, by including a (correct or incorrect) date of birth for that user. An abusive spouse could designate their partner as a child, for instance, and gain some insight into messages being sent on the partner’s phone. Pictures also could be inaccurately flagged as sexually explicit and plan owners would be notified, even though the image being sent or received is something innocuous.
Further, this new Messages filtering feature would likely disproportionately affect LGBTQ+ youth, whose messages about gender or sexuality issues are likely to more often trigger the “sexually explicit” notifications. As some advocates have theorized, this could even lead to unintended outings, abuse from parents, and more.
Scanning Photos Stored in iCloud
The second change that Apple announced involves the detection of potential CSAM images stored in iCloud Photos. Apple’s new feature means that before an image from an Apple device is uploaded to cloud storage, the operating system will use a number of cryptographic techniques to compare the image to the set of known CSAM images that is maintained by the National Center for Missing and Exploited Children (NCMEC). This process takes place partly on the user’s device and partly on Apple’s servers. Because this does not involve scanning users’ image libraries already stored on the company’s server (as competitors’ systems do), Apple argues that this method is more privacy-protective. Images are scanned one-by-one as they are uploaded to iCloud, and the process is done on a user’s device. Apple's new technique enables it to identify iPhone and iPad owners that have CSAM images in their iCloud Photos library, without distributing any CSAM itself and without learning anything about the rest of the images in the device's library. In addition, details of the photos that match the CSAM list are revealed to Apple only after 30 triggering images have been found.
This update marks a significant shift from Apple’s long-held encryption policy and creates a new set of privacy and technical concerns. Under pressure from the FBI after the San Bernardino terrorist attacks to decrypt iPhone user data, Apple pushed back stating that "[f]or many years, we have used encryption to protect our customers’ personal data because we believe it’s the only way to keep their information safe.” Apple has long defended end-to-end encryption in many contexts as the only secure option for its customers, and has touted itself as uniquely protective of user privacy for years, making this shift to on-device content scanning especially remarkable.
Here's an overview of how the new scanning system works:
The first piece is called NeuralHash, which is what is known as a "perceptual hashing" system. Hashing systems are algorithms that take some data (such as an image) and spit out a large number that identifies the input. In most hashing systems, identical inputs will always produce identical outputs. So if I have a file, and you have a copy of that file, they should produce the same hash. Using this technique, you can see if someone else has an image that is in a list of known hashes (such as a CSAM list) by inspecting a hash of the image file and looking in the list for a match. Unfortunately, this also means that changing even one bit in the input data will result in a completely different output. For example, if a user crops or resizes the image, changes colors, or adds a filter, that change will result in a different hash.
Perceptual hashing is different from other kinds of hashing in one important way: different inputs can produce the same output. In other words, two different, but similar, images may produce identical hashes. Apple’s technical summary uses the example of a color image and its greyscale version. While the two files are quite different when you compare their actual bytes, NeuralHash recognizes that they are really the same image, just modified. The same is also true for a number of other transformations. This is intended to prevent people who want to trade CSAM from making small edits to a picture in order to bypass the NCMEC hash list.
An important feature of hashing is that going the other way—from output to input—is impossible. Given the hash from a hashing system, you cannot reproduce the input image, even if you know exactly how the system works. This feature means someone can have a list of image hashes, but cannot use those to recreate the image that generated the hash. That is the principle on which Apple’s multi-step scanning process relies.
Apple’s new process begins with NCMEC (along with any other child protection authorities that Apple allows to contribute to the database, or other government entities that pressure Apple to cooperate) making NeuralHashes from the images in their database of known CSAM. NCMEC will then provide Apple with the CSAM NeuralHash list. Apple encrypts the entries (which Apple refers to as “blinding”) and then places the blinded hashes into a massive table, searchable by NeuralHash. In order to make it harder to guess the hashes of CSAM, Apple fills up the empty spots in the table with random data. Apple then distributes the table to people's devices through an operating system update mechanism, along with the ability to generate NeuralHashes from images on-device.
Using a technique called Private Set Intersection (PSI), the end device runs the NeuralHash algorithm on each image the user adds to their iCloud Photos library. It checks that NeuralHash against the table of blinded CSAM hashes that Apple distributes, and creates an encryption key using whatever data is in the table at the location pointed to by that image’s NeuralHash. The device then uses that key to encrypt a copy of the user’s image along with some metadata including the NeuralHash, to create a “safety voucher.” It then sends that "voucher" to Apple's servers.
The next step takes place on Apple’s servers. Using the NeuralHash of the user’s image provided by the device in the metadata of the voucher, along with the “blinding” key that it used to create the list of hashed images, the server attempts to decrypt the voucher it received from a user’s account. Here, one of two things can happen. If the image included in that voucher matches one of the images on the list of CSAM, then the key created by the end device will match the key created by Apple’s servers, and the voucher will decrypt properly. If, on the other hand, the image is not in the CSAM list, then the matching entry in the original table will have had random numbers in it (remember Apple filled the rest of the table with arbitrary data before sending it out), which the device will have used to encrypt the voucher. Apple will therefore be unable to decrypt the voucher.
Using this system, the client and the server can exchange information about whether a given image possessed by the client is in the set of CSAM images held by the server without sending the set itself to the client or revealing anything else about the client’s images to the server.
The final piece of the process is called Threshold Secret Sharing. This system is used to ensure that, until there are a certain number of positive matches in the PSI system, none of the vouchers will be readable. In this case, until 30 images that match the CSAM hash database are found on a user’s account, Apple cannot review the content of those combined vouchers. Apple is using this technique to make sure the entire system’s false-positive rate is as small as possible. Essentially, if there is some fault in the NeuralHash system and it flags an innocent image as being a CSAM image, Apple still would not be able to view that image unless the same thing occurred for many other images in the same person’s iCloud Photos account. This is achieved by adding another layer of encryption to the voucher system and taking advantage of a technique called Shamir’s Secret Sharing.
Shamir’s Secret Sharing (SSS) is a system that makes it possible to split any piece of data into any number of pieces (called “shares”), and to set how many of those shares are required to reassemble the data. Often used to protect encryption keys, SSS makes it possible to require a certain number of people to agree to a decision, for example, but allow any of the shareholders to make up that number.
In the case of Apple’s system, every iPhone generates its own encryption key during device setup. That key is kept secret on the device, and is used to encrypt the NeuralHash and the copy of the image that’s placed into each voucher. At the same time, the device creates a new share of its secret key, which is included in the voucher’s metadata. The voucher is then encrypted using the PSI scheme described above.
On Apple’s server, the vouchers’ outer layers are decrypted using the PSI scheme, but the inner layer of each voucher is still unreadable due to this new layer of encryption. The server takes the share from the voucher’s metadata, combines it with any other shares it has received from the same device, and attempts to decrypt the inner layer. If the threshold number of matching images has been met for the device in question, the shares will complete the key and ALL of the vouchers from that device will become decryptable.
Once the threshold has been met and Apple can decrypt the vouchers, humans take over. Apple employees review the images that have been included in the vouchers and, if they are determined to be CSAM, the account is disabled and a report is made to NCMEC.
It is unclear how hard it will be to overload this human review system. Apple’s technical summary does not take into account the presence of compromised (hacked) devices, which could upload non-CSAM images that match known CSAM images’ hash. In order to flood the system, someone would need to obtain or reverse-engineer Apple’s server side NeuralHash, which may be possible. As cryptography researcher Sarah Jamie Lewis points out, “given the ease of colliding one kind of perceptual hash it is likely easy to collide a derivative with another.” However, there are enough prerequisites required for such an attack to work, that such an attack still seems like a reasonably remote possibility.
The ultimate privacy and security implications of the CSAM scanning system are not as much with the intentions of the system but rather the choice and transparency over which images’ hashes make it onto the list. Because it would be illegal for Apple to possess the images contained in NCMEC’s database, control over what hashes are included in the list is in the hands of a third-party, and there is not a way for Apple (or anyone else) to meaningfully oversee the content of that database. This makes it hard (impossible even?) for Apple to be transparent about what images are being scanned for and requires a certain level of trust in the partner organization who develops and maintains the hash database. This is not an argument about whether or not to trust the hashes provided by NCMEC, but simply an observation that Apple has built the technical machinery to scan for "bad things" based on a list it does not control and cannot audit. The safeguard against inserting non-CSAM hashes into the table is the human filtering system, but the technical parts of the scanning system would still work if the human filtering step gets removed from the process.
Apple is planning to roll the system out with the upcoming iOS 15, iPad OS 15, WatchOS 8 software updates, and at this time the features will only be applied to U.S. users. However, governments around the world will likely demand that Apple apply these features in other jurisdictions, and for other types of hashes, now that the technology exists. Apple has promised that it will refuse requests from any other entities that demand access, and pointed out that it has done so in the past. However, the delicate balance of Apple’s relationship with governments around the world may have shifted in ways that put user privacy and security at risk. By creating this system, Apple has transformed what have always been large scale demands—such as circumventing the encryption on the iPhone in a way that has never been done before—into a much smaller change by simply adding these additional images to an already existing filtering system.
Much like the Messages image detection tool, once Apple builds in the ability for specific “types” of content to be scanned for on users’ devices, governments will likely want to use that capability to identify other types of content, and will pressure Apple to do so. However, unlike the new Messages feature, where only senders and recipients see the notification, hash matches for uploaded photos will be shared with law enforcement or other government actors. This could be used to conduct political targeting and surveillance, and identification of certain types of images could result in serious legal consequences, especially for vulnerable groups. For example, in countries where being LGBTQ+ is illegal, just uploading images containing a rainbow flag could lead to imprisonment or worse.
Notably, photos stored via iCloud Photos are currently not encrypted when saved on Apple’s servers (which would ensure that Apple could not view the data). This fact is significant because there isn’t a technical reason Apple couldn’t currently review users’ photos that have been uploaded to its own servers. Scanning unencrypted files stored in the cloud is the approach taken by other companies, including Dropbox, Google, and Microsoft (who have their own methods for these processes). Instead of using similar server-side scanning practices as its peers, Apple will be scanning directly on individuals’ devices. Apple therefore designed this new scanning system to work in an environment in which Apple doesn’t have to see the content of the images being scanned—why remains an open question.
Apple's different approach is important because the intersection of end-to-end encrypted messaging and CSAM has been a technology flashpoint for years now. Prior to Apple introducing its system, many cryptographers and tech policy experts had argued that building a system to scan personal images for CSAM would be dangerous because of the direct privacy implications as well as the potential for abuse that it presented. Apple’s solution takes some steps to address the privacy implications, but the potential for abuse of this system is still just as concerning.
Changes to Siri and Search Results
The third change that Apple announced, and the one with likely the least impact on user privacy and security, is in the way that Siri and Search respond to searches for and about CSAM. Searches about how a person can report CSAM or child exploitation will direct the user to resources for help making a report. Searches related to CSAM itself will receive a response saying that interest in this topic is harmful and the user will be directed to resources to get help. While this change will affect the responses people get from Siri and Search, Apple has not announced any changes to its collection of data about these searches and so no particular privacy implications are apparent.
Conclusion
Ultimately, Apple’s new features raise significant privacy and security concerns—specifically the changes to Messages and the new scanning process for CSAM in iCloud Photos. These concerns include whether the technical capability to implement these features while protecting user privacy is possible, and whether Apple will be able to meaningfully push back on attempts of outside actors to co-opt these features for their own ends. While these features will affect all users, they may disproportionately harm at-risk communities such as the LGBTQ+ community, journalists, and political dissidents, especially if these features are turned into a mass surveillance tool. We will continue to monitor and work to combat the privacy and security implications these features introduce.