When Students Get Lost in the Algorithm: The Problems with Nevada's AI School Funding Experiment
Blog Post
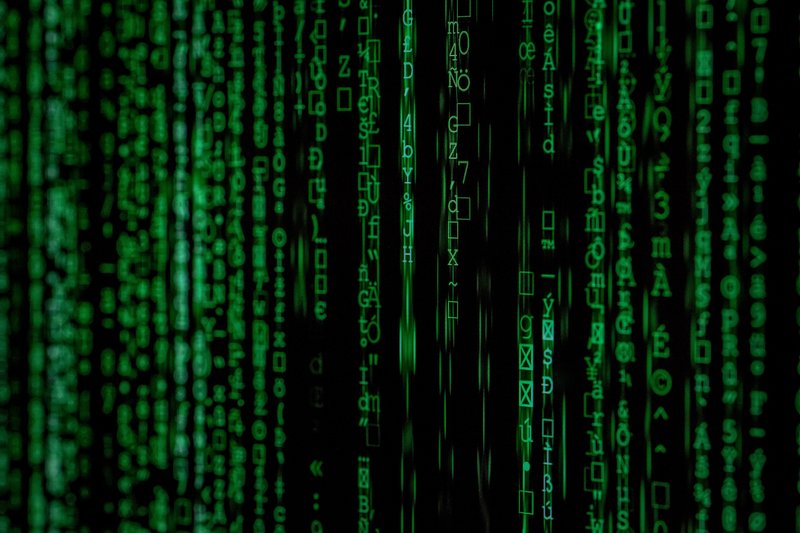
Marlus Spiske on Unsplash
April 3, 2024
In its 2023 education budget, Nevada fundamentally changed the way it provides funding for students with higher needs. Where before it used family income data to determine if a student is considered to be “at-risk” and therefore eligible for greater funding, it now uses a machine learning-based predictive model that attempts to identify students at the greatest risk of failing to graduate.
Developed by third-party contractor Infinite Campus, the new model uses 75 inputs, including a student's grades, standardized test scores, and attendance data to generate a “grad score” for each public school student. It does still take into account whether a student comes from a low-income household, but this is only one indicator alongside 74 others that have nothing to do with economic status. This new policy reflects a choice to allocate at-risk funding based not on economic need, but on a compound metric that is far less clearly defined. This change moves the state away from broadly supporting students in poverty and towards a funding policy that, despite its use of many more data points, is neither transparent nor better targeted.
The grad scores—which Infinite Campus’ own CEO has likened to a credit score—range from 50 to 150. A cutoff point of 72 determines whether the state will provide additional aid. This new way of defining “at-risk” students reduced the number who were eligible for supplemental state funding from 288,000 in the 2022-23 school year to only 63,000 by the following year. But the needs of the state’s at-risk students didn’t change overnight—only the methods used to identify them.
But the needs of the state’s at-risk students didn’t change overnight—only the methods used to identify them.
State officials praise the new system’s ability to target funds to the students the model decides have the highest needs, but the model was constructed with little transparency, raising questions about whether these students are, in fact, the ones who require the most support. Though the list of variables used to create grad scores is publicly available, the weights used to generate the scores are not. Without knowing exactly how these scores are calculated, there is no way to meaningfully evaluate whether the model really identifies the students with the highest levels of need.
Without knowing exactly how these scores are calculated, there is no way to meaningfully evaluate whether the model really identifies the students with the highest levels of need.
Meanwhile, despite overwhelming evidence supporting the greater funding needs of students from low-income backgrounds, grad scores systematically deemphasize economic status. Nevada changed its approach in order to target a narrower group of high-needs students, but the new model risks leaving many high-poverty schools short of the funds needed to provide their students with essential support.
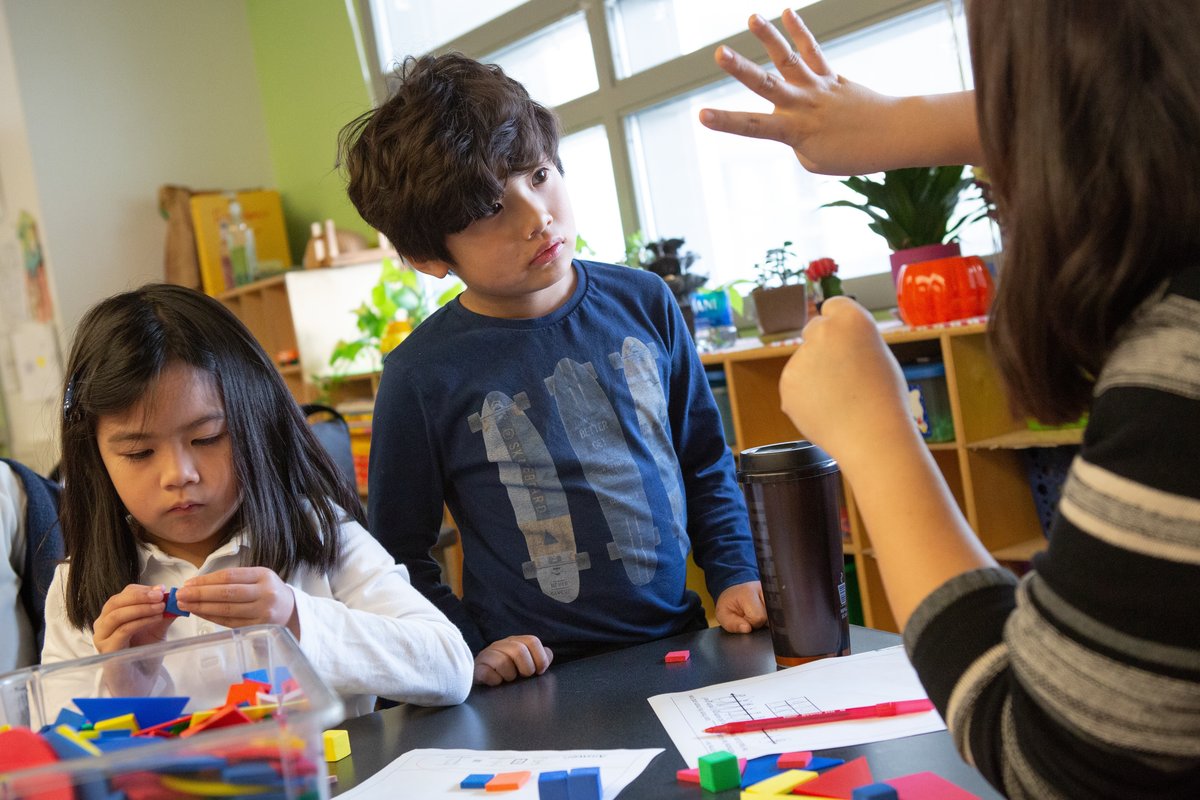
Source: Photo by Allison Shelley for EDUimages
While it’s too soon to fully evaluate the impact of this policy change, we do know hundreds of thousands of low-income students across the state are no longer receiving supplemental aid. These students have demonstrably greater funding needs than those from higher-income families, and this model is responsible for taking that critical funding away. Predictive analytics and artificial intelligence are powerful tools that have the potential to help administrators create innovative ways to target students who need support, but they shouldn’t be used to direct funding away from low-income students as a group. They can’t be used to play shell games with the resources intended for students that we already know are vulnerable.
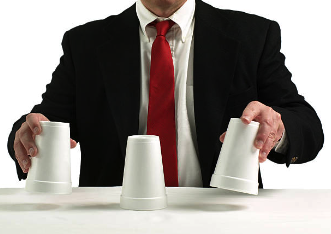
Source: Aberenyi on iStock
Nevada avoided reducing total funding for at-risk students in the first budget year of the new system, so for now, fewer students are each receiving more funding. But it’s not hard to imagine a scenario where the grad score model could be used as political cover to justify slashing state education budgets, either in future years or under similar systems in other states.
A more data-rich model should allow for more nuance in how students are funded, but the grad score still relies on a simple eligibility threshold: students get a flat amount of funding if they score above 72 on an opaquely defined scale, and no supplemental funding if they don’t. This binary cut-off is no more sophisticated than the basic, income-level-based method it replaced; it simply relies on different inputs. Why not envision a system that truly takes advantage of the wealth of data at the state’s disposal? One that provides some level of supplemental funding to all low-income students but is still able to take their differing levels of need into account?. Instead of using grad scores as a cut-off point to determine at-risk status, cutting this aid for 75 percent of formerly eligible students, a new model could just as easily provide funding on a sliding scale, with the highest needs students receiving the most additional funds. Indeed, Texas's school funding system already uses data from the U.S. Census to send greater funding to low-income students from higher-need communities.
Why not envision a system that truly takes advantage of the wealth of data at the state’s disposal?
Nevada is not going to be the last state to integrate big data and predictive analytics into its school funding formula, so we need to think critically about how best to deploy these tools. There is value in efforts to use these resources to better target high-need students, and education funding models can certainly take full advantage of the growing data resources available. However, if school funding—and by extension, the opportunities available to students—are directly linked to the outputs of a machine learning model, then that model must be designed with transparency, equity, and accountability in mind from the start. The methodology must be in the public domain, so that they can be evaluated for how fairly and well they actually support high-need students–which should include students from low-income backgrounds as well as those with other needs and challenges. Nevada’s new policy falls short on all these fronts, leaving too many students out of the equation.