The Automation of Admissions: Predictive Analytics Use in Enrollment Management
1 of 2 Blog Series
Blog Post
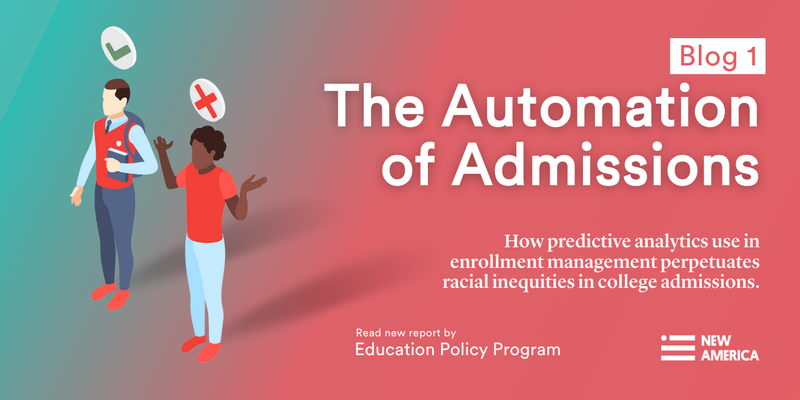
Oct. 6, 2021
This is the first post in a blog series that raises awareness of how algorithmic biases in enrollment management decrease access for Black and brown students. In this series, we demystify the process of college admissions and provide recommendations for institutions. You can see our second post here. Please also view our short video to highlight some of the equity concerns we raise in the blog series to show how the automation of admissions perpetuates racial inequities to college access.
When most of us think of college admissions we picture well-meaning administrators reviewing piles of application forms and sending acceptance letters to those who are most qualified. But there is a hidden side to admissions fewer people are aware of: enrollment management.
Enrollment management is the behind-the-scenes of the admissions world, and it is increasingly automated with the use of predictive analytics. The problem is that with the automation of enrollment management comes the perpetuation of racial inequities in college admissions.
In order to understand how the automation of admissions perpetuates systemic racism, we have to demystify the enrollment management process. At many colleges, a team works together to build a near-perfect class that meets institutional goals. This team varies in size depending on institutional resources, but it can be composed of admissions officers, institutional leadership, institutional researchers, and, often, private contractors.
Together, enrollment managers funnel students through five phases to get them to apply and enroll at their institution: recruit, apply, admit, deposit, and enroll. At the recruitment phase, enrollment managers work to round up as many potential applicants as possible. Then, they work to get these students to submit a completed application and proceed through the well-known admissions process. Finally, after review of the applications, the college sends acceptance letters, and students can choose to accept by submitting a deposit as a sign of their intent to enroll in the upcoming fall.
This process leads to the most important part of the cycle for colleges: yield. Yield is the number of students who put down a deposit and show up for the first day of class out of all the students the college recruited throughout the admissions process. In many ways, yield represents how well the enrollment management team performed that year in meeting its goal number for students in the first-year class. Throughout this entire process, enrollment managers focus their efforts on students who, with a little nudge, are most likely to yield.
Enrollment management is a very human and labor-intensive job, so it has grown to rely on data and predictive analytics over the past 20 years to help do the job better. But as with any other use of predictive analytics, it is imperfect, where biases and inequities can easily creep in. And without the human element, these biases could go unchecked.
And that is where the problem is, the unchecked automation of enrollment management and admissions. There is an increasing body of work criticizing enrollment management, but few pay attention to the racial equity issues that arise from the automation of admissions through data and predictive analytics.
In our brief video we explain how enrollment managers use data like SAT and ACT scores, zip code, grades, and even whether a student has taken a campus tour, to build the algorithms that predict yield. The problem is that data are a numerical representation of our society. And since systemic racism permeates every part of our society, including the K–12 and higher education systems, solely using data and predictive analytics without considering the humanity of students to make decisions about who gets in and who does not runs the risk of perpetuating it.
Take campus tours, for example. This is a seemingly innocent data point, but it puts students of color, who are often low-income and first-generation, at a disadvantage. Campus tours are used to represent demonstrated interest, so if a student visits and goes on a campus tour, the college could use the information the student used to sign up for the tour to measure his or her demonstrated interest. This could work in the student's benefit at application time, as the college now sees that the student is interested and more likely to yield.
But campus tours are not easily accessible by everyone. Many students do not know that campus tours exist and that they can, and maybe should, attend one. Other students may know that tours exist but not be able to afford to make the trip. Yet other students cannot afford to take the time to take a tour, even if a campus is close by. They may be busy working and taking care of family responsibilities.
These kinds of seemingly innocent data points are plugged into a predictive model to help colleges decide who gets into a four-year college, and who does not. And without the human element to serve as a potential checkpoint for automated biases, students of color could be incorrectly identified as unlikely to yield and be less likely to receive an admission decision. Depending solely on predictive models raises the risk of data and analytics perpetuating systemic racism.
These are the types of racial inequities that are perpetuated with the use of predictive analytics in enrollment management. When colleges primarily rely on predictive analytics in enrollment management, they are handing over life-changing decisions to machines. If institutions do not use caution in the automation of admissions and consider the human element during the admissions process, they risk continuing to perpetuate the racial equity gaps in access to college and a better life for Black and brown students.
Enjoy what you read? Subscribe to our newsletter to receive updates on what’s new in Education Policy!