Why Numbers can be Neutral but Data Can’t
Blog Post
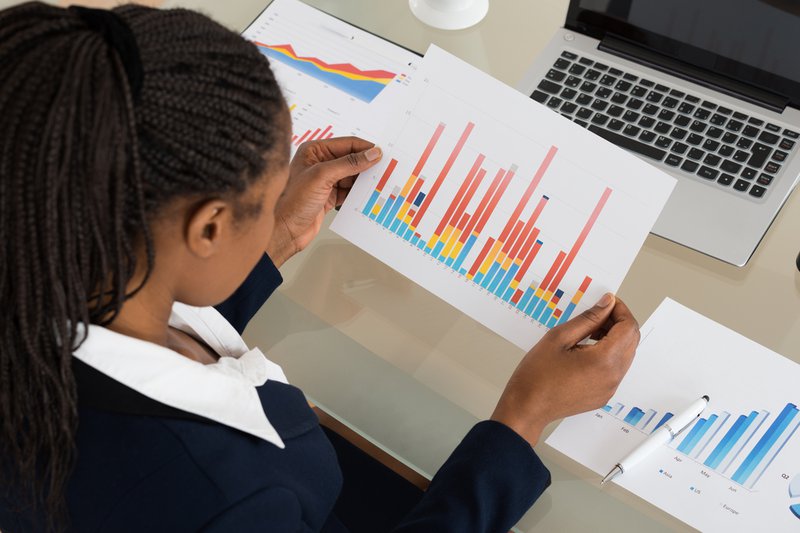
April 29, 2016
This month is Math Awareness Month (MAM). Celebrated every April since 1986, MAM seeks to increase the visibility of mathematics as a field of study as well as the public’s understanding of and appreciation for math. With a different theme chosen each year, this year’s theme was the future of prediction: exploring how mathematics and statistics enable us to make predictions--about the weather, the spread of disease, and even which students are at-risk of not succeeding in college. This year’s celebration also asked participants to contemplate the role math will play in uncovering novel predictions in the years to come.
Math and numbers are ubiquitous in our daily lives. For example, figuring out if it’s cheaper to order takeout or prepare a meal at home or calculating how much to leave for a 15 percent tip. This might be why it’s so easy to conflate mathematics, and its inherent use of numbers with data about people. A number is an arithmetical value, expressed by as a word, symbol, or figure, representing a particular quantity. Data, on the other hand, is information expressed as numbers. And, numbers and math are related as mathematics is the abstract science of numbers, quantity, and space.
Our use of “big data,” or extremely large data sets, has only added to a numbers-driven world. Netflix and Amazon are examples of two companies that use big data to market their products to user’s individual interests. MAM dedicating its 2012 theme to big data in our everyday lives--both the opportunities and dangers--speaks to this phenomenon. Higher education has not been immune to this trend. In fact, the field has been dominated by discussions about how big data can be best utilized to improve outcomes for institutions and other stakeholders and help students meet their educational goals.
Despite being an avid proponent of using and appreciating mathematics--with virtually every college campus offering a major or minor in mathematics, and mathematics being a requirement to complete most academic programs--higher education administrators, faculty, advisors, and consultants aren’t exempt from this proclivity to confuse mathematics, numbers, and data. This is especially true in how the field communicates these concepts. A data awareness month might be useful if it helped higher education parse out the key differences among them.
Poor Communication
Data is often referred to and seen as neutral. It is also believed that it’s how data is used that matters, for example using student data to ensure students--or bunnies--swim and not drown. A similar sentiment has been said about education technology, which often requires using or gathering student data to ensure their success. Take for instance Condoleezza Rice stating at a recent edtech conference that:
"Technology is neutral. It’s not good or bad. It’s how it is applied that matters.”
The truth is, data--and education technology for that matter--are not and cannot be neutral. While often presented as numbers, and derived from applied mathematics--such as statistics--data are not synonymous with numbers or with math. While a data scientist, analyst, or statistician can make computations using data (applied mathematics) and present data about people as numbers (a mathematical symbol or object created in abstraction), this doesn’t make data about people neutral. What differentiates data from numbers is that numbers are mathematical abstractions, an idea. Because numbers are symbols or objects used in math, they can be neutral. But data, originating from the real world and real people, cannot.
Higher education administrators, faculty, advisors, and consultants poorly communicate what data is, and how applied mathematics--like statistics--are helpful in analyzing data to solve or understand problems in higher education. Attempting to simplify education jargon, and the term data-driven in particular, an NPR article highlighted its use of a text editor that restricts you to the 1,000 most common words in the English language. The resulting definition for data-driven was:
“We should decide things using numbers.”
Here again data and numbers are made to be synonymous when they are not. While it may seem insignificant, any attempt to codify commonly-used language impacts the way we understand and communicate what we as practitioners, policy makers, etc. do. Thus, any effort made with faulty understanding and using loose diction can be detrimental.
As a final example, describing how using data propelled a university to realize it needed to change its advising strategy, one administrator conflates numbers and data. He reportedly stated:
“All of a sudden we’re talking about real numbers.”
It’s unclear if unreal numbers even exist. Imaginary numbers, yes. But unreal numbers when those numbers represent students? It’s more likely that what was meant by real was that the data was objective, neutral. But, as I’ve said before, this isn’t possible.
The power of data in higher education isn’t what’s in question: using data appropriately can result in impressive gains for a college and its students. This same college saw their fall-to-spring retention for first-time freshmen and sophomores surpass 90 percent, an increase of 3.4 percentage points from the previous year. However, precisely because of its power and promise to help institutions move the needle on student success, is why fully understanding what data is and properly communicating about it is essential.
Remedy 1: Rethink Data
How can we avoid conceptualizing and communicating about data as an abstraction, purely numerical in nature? One way is to acknowledge how when we often communicate and share numbers, we really are talking about data on people, systems, and norms, none of which are abstractions or neutral. A way of thinking about data in this way can be best summarized in Acumen’s, a non-profit that raises charitable donations to invest in companies, leaders, and ideas that are changing the way the world tackles poverty, discussion about using data to measure social impact. The authors state:
“It’s all too easy to forget that data is about human beings and their behaviors. Data is not an abstraction. The social development sector is prone to forgetting this. We often collect data with little regard for the people behind the numbers.”
“Data encodes the stories of our lives, capturing not only our tastes and interests but also our hopes and fears. Data isn’t an abstract idea or a set of numbers or qualitative responses. It can be and is, ultimately, human.”
In a similar vein, at a recent forum on the reliance on data-driven risk assessments by governments and the human rights implications of this trend, Helen Nissenbaum, a professor at New York University, stated:
“We talk of data as if it’s raw fuel of algorithmic analysis. It's collected with a purpose and not unbiased.”
Although the discussion was about the implications of predictive analytics use for human rights, this same caution can and should be applied to higher education. This is especially true as the use of predictive analytics and other algorithmic-based tools continue to gain momentum in academia.
Experts working in education have also expressed similar views. For example, Mimi Onuoha, a fellow at Data & Society, a research institute focused on social, cultural, and ethical issues arising from data-centric technological development wrote that:
“Every data set involving people implies subjects and objects, those who collect and those who make up the collected. It is imperative to remember that on both sides we have human beings."
Mikaela Pitcan, a research analyst at Data & Society pointedly wrote:
“The assumption of “objective data” frees people from acknowledging structural inequality.”
Has the field asked directly what the underlying forces are that enable higher education to be the best equipped to understand and yet possibly more inclined to forget that the common denominator in data are people?
Remedy 2: Rethink Math Education
In addition to a new way of thinking about data, higher education might also need to take seriously the appeals to make math undergraduate education more applicable to real-world problems. By doing so, we might not only be able to reduce math as an early stumbling block for students in their college careers, but equip the next generation of leaders to have a more nuanced understanding and communication of what data is, where it comes from, and ways to use and analyze it. This includes the many ways we can mishandle data at each stage.
There have been national calls to make math more relevant and overhaul how it is taught on college campuses--away from the abstract to the practical. Transforming Post-Secondary Education in Mathematics (TPSE Math), a project by nationally recognized mathematics education leaders formed in 2011 to push for this change. Among the things called for, is an entry-level math course that is relevant for the career goals and interests of every student at every college.
TPSE Math isn’t alone however in their calls for math to not only be better taught, but teach relevant skills students will need to contribute to solving real-world problems. Andrew Hacker, a professor emeritus at Queens College of the City University of New York, makes the distinction between math and arithmetic and says that colleges should focus on teaching better and upgrading the latter. Math means algebra, trigonometry, and calculus, all part of what he calls the "enigmatic orbit of abstractions.” And for Hacker, arithmetic is the quantitative literacy that people actually need. Hacker has gone as far as to say that students, educators, and the like should learn to be skeptical about numbers, especially when they’re situated in the real world. This dovetails with many others understanding that data has its imperfections, one being its non-neutral nature.
Remedy 3: Convene and Train
Changing the way math is taught at the undergraduate level is a policy change that will understandably take, among other things: time, effort, resources, organizing of key stakeholders, and political will. At the very least however, the field could devise ways to bring institutional leaders, policymakers, and anyone who makes decisions using data to engage in conversation and trainings on the true essence of data--where it comes from, and the ways it’s analyzed. The goal would be to help the field remember that data doesn’t exist in the abstract, but in the real world.
An initial step could even be to critically examine what others--outlined earlier--have already said on the subject. And ultimately, convenings and trainings could keep our conversations about data grounded closer to its origin: among people, institutions, systems, norms, and values. We might then be able to strengthen our understanding, communication, and transparency around how we collect, analyze, interpret, and communicate data with these same people, processes, and structures in mind."