Coronavirus Throws Predictive Algorithms Out for a Loop
The data predictive algorithms depend on has changed due to the pandemic.
Blog Post
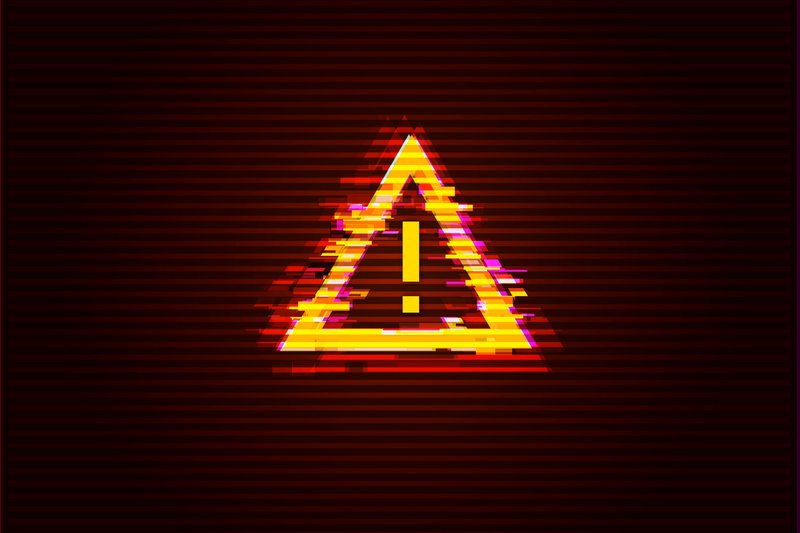
Shutterstock
May 4, 2020
Predictive analytics has empowered institutions to predict student outcomes like enrollment and retention and even identify the factors that are likely to lead to, or derail, these outcomes. A student’s choice to enroll in an institution, for instance, could be predicted by factors as specific as student interaction with school websites. And retaining a student can be forecast based on GPA or location data.
All of these predictions were based on patterns found in existing data on students' likelihood to pick a college or persist to graduation -- patterns that determined what a predictive model looked like. But none of what is happening in higher education as a consequence of the coronavirus pandemic has happened before. Among all of the other chaos it has caused, the pandemic has thrown the use of predictive analytics in higher education out for a loop.
Here’s how it normally works: institutions use data sets that span various years and look for patterns in that data. Particular variables that have strong predictive capability of an outcome are strung together to make a predictive model. Then, data is fed through the model and a predicted outcome is generated. The desired outcome can be, in the most general sense, the likelihood of enrolling at an institution or likelihood of graduation.
But in the case of a pandemic, none of the current behaviors have occured before, and that means the existing predictive models no longer work. The data fed into the models may be different, or even no longer exist, and what these models were predicting before the pandemic likely doesn’t apply to the current reality.
Take the changes that have happened in the college search and admissions process this year. Many of the data points enrollment managers and institutions used to predict a student’s likelihood of enrolling have completely changed. For example, at some institutions, whether or not a student attended a campus tour has a strong predictive power for enrollment. But with state stay at home orders and institutions closing campuses to minimize the spread of COVID-19, many Spring semester campus tours were moved online or cancelled completely. It is unknown whether attendance at an online campus visit or yield event has the same predictive power as an in person campus visit and how lack of visits could affect enrollment- there is no existing data or pattern for that. This change has made that data point virtually useless to enrollment managers, and has thrown off the entire predictive model itself too.
Another data point enrollment managers depend on is a student’s Expected Family Contribution (EFC). This data point and other related financial data can help institutions determine the amount of institutional financial aid necessary to virtually guarantee a student’s enrollment in the institution. But so many families’ financial situations have changed as a result of the jobs lost during the coronavirus pandemic (at the same time that institutions have also lost a lot of money), so this information is not as predictive of enrollment as it was just last year. The pandemic has changed these normally predictive factors, as no prior data exists on how students select an institution during a pandemic, and have made enrollment managers’ ability to somewhat depend on prior patterns to predict Fall semester enrollment virtually impossible.
On the retention side, the challenges are similar. Retention predictive models tend to use data points such as engagement with counselors, grades, and online learning management system engagement data to predict a student’s likelihood of graduating or risk of dropping out. But these data points have also completely changed in light of higher education going fully online during the pandemic. Access to counselors has changed at many institutions -- some have been able to quickly and successfully go online, while others are still struggling to deliver a similar quality service. Many institutions or courses have adopted pass/no pass policies, which could affect how a predictive model calculates how student letter grades affect risk scores. And the way students engage with learning management systems, such as Canvas, are undoubtedly different than how they did before the pandemic, whether that be for better or worse. These and many other likely unaccounted for external factors will affect a student’s likelihood of graduating and will make the accuracy of an existing retention focused predictive model shift significantly.
The power of predictive analytics in higher education is real, but the coronavirus pandemic has made it clear that predictive analytics can’t predict the future, it can only forecast an event based on what has already happened before. How institutions adapt their algorithms and enrollment and retention practices in light of the pandemic will be an interesting and important development to watch. And while the pandemic has thrown predictive analytics in higher education (and many, many other things) out for a loop, this may also be an opportunity to re-evaluate predictive models, policies, and practices to ensure they are as equitable and accurate as possible.
Interested in staying up to date on education and workforce policy? Subscribe to our newsletter to receive updates on the latest from our experts.