Grounding Principles for Understanding and Regulating AI
A public interest technologist offers a high-level framework for how to sift through the hype surrounding generative AI, make informed personal and organizational decisions, and hold this new iteration of technology accountable.
Blog Post
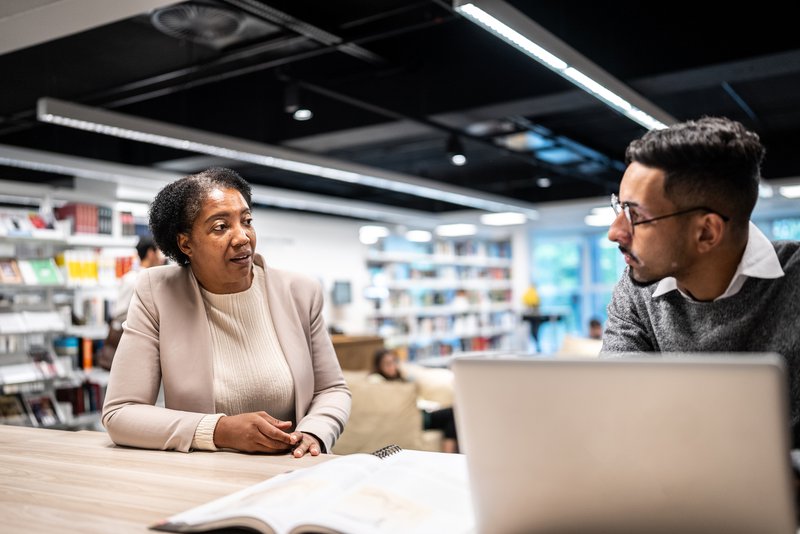
FG Trade, via iStock.com
May 18, 2023
Maria Filippelli is the Data Director for the Southern Economic Advancement Project, and a former Public Interest Technology Census Fellow with New America. As a PIT Fellow, she developed and led a strategy to assist dozens of national, state, and local organizations and governments navigate the technical changes to the 2020 Census.
A few weeks ago my yoga instructor asked me after class about the hype surrounding ChatGPT and generative AI. Did I think it really was a watershed moment in humanity? It was early in the day, and my immediate response was that only history can determine if this is a watershed moment. However, I added, the actions we take now to understand and weigh the pros and cons of generative AI are incredibly important.
He nodded thoughtfully, and seemed to be gathering his thoughts for a follow-up question, but it didn’t come. As the day wore on, I realized that my answer was clear but probably insufficient. My yoga instructor wasn’t really looking for a single answer; he was, like many of us, looking for a framework to sort through the immense swirl of claims, counterclaims, hype, and critique about generative AI that has been unleashed since ChatGPT’s release in November 2022.
Read the May PITUNiverse Newsletter on Data Science & AI
And I realized that I hadn’t seen much in the way of useful frameworks for experts and nonexperts alike to evaluate generative AI products. As a public interest technology practitioner who has worked on multimodal trip planners, open data portals, and emerging tech issues surrounding the 2020 Census, I was evaluating everything I was reading much in the same way as any other PIT project I’ve worked on: understanding the problem to solve, investigating the data involved, and pressure testing my ideas with stakeholders who will challenge my thinking.
The articles I’ve read, webinars I’ve watched, and conversations I’ve been a part of run quite the gambit, from “we must stop all generative AI development now” to “generative AI will make its way into every aspect of our lives.” After explaining how AI works in simple terms, they either propose and hype specific solutions – or discuss concerns about particular aspects of generative AI like problematic training data, mis- and disinformation, algorithmic “black boxes,” and the acceleration of data-powered racism, sexism, ableism, and other forms of bias.
What seems like a solution to perform a task faster can often lead to downstream problems.
As I reflect on the recent PIT-UN webinar with Meredith Broussard (NYU), Todd Richmond (Pardee RAND), and Vanessa Parli (Stanford) on Higher Education & Generative AI and ponder the conversations I’ve had with my yoga instructor, family, friends, and colleagues, my own PIT framework for evaluating generative AI came into focus. Most frameworks focus on the algorithms themselves. But, like PIT-UN Member Suresh Venkatasubramanian (Brown) , I recommend focusing not on the particulars of any one technology or two dominating the headlines, but on the high-level questions we can ask of any technology. (Consider that this time last year, conversations about tech were focused on blockchain and cryptocurrencies; the year before, they were all about COVID-19 contact tracing apps; I could go on…)
Below, I offer a high-level framework for how to sift through the hype surrounding generative AI, make informed personal and organizational decisions about how to use (or not use) generative AI tools, and how we as a society can hold this new iteration of technology accountable going forward.
Understand the Problem: Why was this product created?
The first step in any public interest technology project is to understand the problem you’re trying to solve. Too many tech tools are created without fully understanding the problem or taking the time to get to the root cause (Power to the Public shares many examples of this). What seems like a solution to perform a task faster and with less friction can often lead to downstream problems, from waiting longer in TSA lines to disinformation to automating inequality.
The same principle holds true for generative AI: What problem is it trying to solve, and what problem are you, as an individual or organization, trying to solve?
For example, there is a company developing a solution for doctors to use generative AI to determine patient care plans. The justification for this solution is that doctors are busy. OK, that’s true. But if we really dive into why doctors are busy, we find deeper root problems like patient loads and other demands on their time. So, is developing a patient care plan with AI really a solution, or just an imperfect Band-Aid on a very complex and poorly designed medical system? An automated, error-prone, and opaque technology is probably not the best solution to this multilayered problem.
We should focus not on the particulars of any one technology dominating headlines, but on the high-level questions we can ask of any technology
As more generative AI applications come to market, you will certainly be hearing from vendors about them. You might even feel pressure from your supervisors or institutional leaders to adopt these tools so as to keep pace with the rate of technological change. Here are some things to keep in mind while navigating these conversations:
- Who was involved in the development? Was a wide range of stakeholders with a diversity of lived experiences consulted for feedback on design?
- How has the vendor addressed unintended consequences? Is there a record of stress testing the tool outside of the intended use? Where is that documented?
- What is the data stewardship plan? What data about you and your interactions with the tool will be recorded? How will that data be used? Who will it be shared with? Can you opt out of all data tracking and selling?
Know the Data: What is informing the AI?
Research has long shown that the algorithms that power machine learning and AI are biased, because the data used to develop and train these systems reflects our society’s biases. There’s a body of work discussing how this happens in every sector, from automated decision making in hiring to pretrial risk assessments in the justice system.
This was one of the key points Meredith Broussard made in the recent PIT-UN webinar. She noted that toxic training data and indiscriminate web-scraping lead generative AI tools to merely reiterate, but with more confidence, the contradictory mix of truth and lies, valuable insight and slanderous hate speech evident in existing online spaces such as Reddit.
Meredith’s critique of training data is complex and multilayered — I encourage you to review her many articles and two books on the topic — and point us to the following fundamental questions about data:
- How was it collected: through web scraping, surveys, and the like?
- What was the original purpose? (Utilizing data for a different purpose than originally intended can skew results.)
- Is there hate speech in the data? (Spoiler, it is.)
- Was this data privacy protected? Clearview AI was fined by multiple countries for using people’s images to train AI models without their knowledge
The training data sources and weights are one reason I question the validity of AI. It takes inputs and returns generalizations, and it has historically been difficult to find any specificity in outputs. For example, the National League of Cities recently asked ChatGPT about its use for local governments. The responses reiterated jargon like improved data-driven decision making and providing insights. As a former urban planner, I am underwhelmed by the conversation. Applying a digital product with such potential impact requires that a local government not have any gaps in the digital divide to ensure equity and that it have machine-readable documents to train the system, among other considerations. These are difficult hurdles for any government.
There is No Substitute for Transparency: How is the AI built?
After understanding the problem to be solved and the data used to create generative AI, we need to ask how the tools are actually built. Advocates have long been asking for that in social media ads and to protect civil rights.
Transparency is a function of technology governance. Given that businesses in a hypercompetitive capitalist system are incentivized to keep their systems secret from regulators and competitors, transparency will become an industry standard only through top-down regulation. Facebook’s Mark Zuckerberg essentially asks for regulation in his 2020 congressional testimony when he states, “We stand ready to work with Congress on what regulation could look like.” Just this week, Sam Altman of OpenAI said much the same. Big Tech has shown us over and over that they prioritize global solutions and profits over altruistic actions.
Read the Blueprint for an AI Bill of Rights
The Blueprint for an AI Bill of Rights , a research-based set of guidelines developed by the Biden Administration’s Office of Science and Technology Policy, outlines some key principles for developing such legislation. Companies should be required to “describe, in plain language, how the system works and how any automated component is used to determine an action or decision. It should also include expectations about reporting…such as the algorithmic impact assessments described as part of Algorithmic Discrimination Protections.” Implementing these plans is crucial to protect civil rights and preserve our privacy.
If you’re not directly responsible for implementing an AI tool, the blueprint principles can be referenced when you’re assessing whether to download an app. Consider if the “terms & agreement” language is clear and easy to understand, if you know what will happen when an incident occurs, and how you can opt out of having your data collected and shared.
Let’s Talk About It
During my census work, a colleague once told me that I was talking to the wrong people. They didn’t have a clear response when I asked who the right people were. But the sentiment was clear: Some people are worth bringing into a conversation, and some people are not.
This type of thinking cannot continue. The ubiquitousness of technology, and the rate at which generative AI tools are hitting the market, demands that we include as many people as possible in our decision making.
We are asked to give our personal information for commercial reasons — when signing up for an account on social media, when purchasing something online, even to read an online article. We are also asked to give our personal information for government uses and health care apps. We show up in lots of data sets, and we deserve to know how our data is being used. For too long, questions of technology design, deployment, and governance have been seen as the provenance of those developing the technology. It’s time for us, experts and nonexperts, to ask more questions of vendors and engage in discourse more often.
Many pieces on generative AI, and this one is no exception, reference the explosion of conversations around AI. This is a good thing, so long as the conversations are focused on the right questions. This is perhaps the biggest contribution that PIT professionals can make: articulating and arguing for the right set of questions and the right frameworks that will help individuals, collectives, and society writ large shape the course of generative AI’s development, deployment, and governance.
Discussions and interrogations into why a tool was developed and what data was used for it will help hold tech companies accountable. And if we continue to have conversations among ourselves and with our yoga instructors about pros and cons of generative AI, we’re moving in the right direction.
Note: This article was researched and written completely by a human. No generative AI solution was used in its production.
This piece is part of the May 2023 PITUNiverse newsletter on Data Science & AI. Subscribe to get thought leadership, resources and opportunities from across the Public Interest Technology University Network straight to your inbox.