Regulating Platform Algorithms
Approaches for EU and U.S. Policymakers
Brief
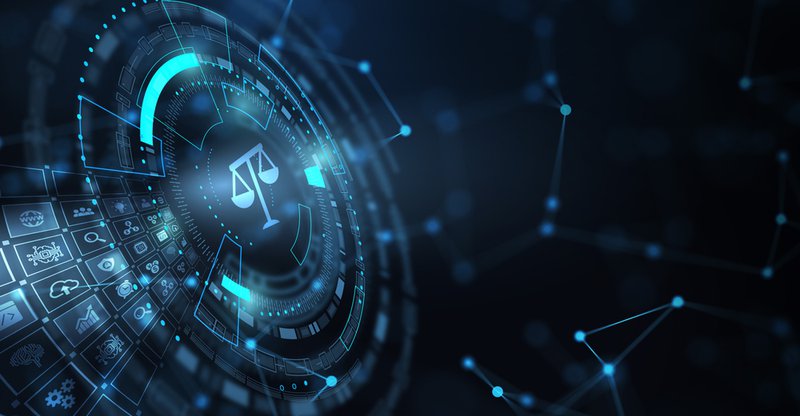
Photon photo/shutterstock.com
Dec. 1, 2021
Introduction
Internet platforms rely on artificial intelligence (AI) and machine learning (ML)-based tools to curate the content we see online. Platforms particularly use automated tools for content moderation, ad targeting and delivery, and content ranking and recommendation. While these methods enable platforms to create personalized user experiences at scale, they can also generate harmful and discriminatory outcomes. Despite continuous advocacy efforts to obtain transparency and accountability around the development, use, and impact of these systems, platforms are sorely lacking in this regard. Additionally, platforms’ current default practice of self-regulation amounts to very little accountability and significant real-world harm. As a result, policymakers are exploring legislation to promote algorithmic accountability.
In the European Union (EU), policymakers have tackled the issue of algorithmic regulation and accountability by introducing two comprehensive legislative proposals. The first proposal—the Digital Services Act (DSA)—outlines obligations internet platforms must meet when removing illegal and harmful content from their services. The DSA also requires platforms to provide transparency around their content moderation and algorithmic content curation efforts. The second proposal, the Artificial Intelligence Act (AI Act), uses a risk-based framework to categorize certain AI systems as “high-risk.” Under the AI Act, certain systems posing too significant of a risk to fundamental rights would be prohibited. Other high-risk AI systems would be permitted but subject to prescribed safeguards. The AI Act applies to various sectors, including employment, law enforcement, and internet platforms. Not all provisions in the proposal may apply to internet platforms, however. The EU Commission must provide greater clarity around which ones do.
U.S. policymakers have approached algorithmic regulation through a patchwork of potential solutions that vary in their granularity and focus. Designing meaningful regulation, particularly when it comes to algorithmic systems that curate online speech, has proven challenging as proposed rules must comport with the First Amendment. Under the First Amendment, the U.S. government cannot require platforms to keep or remove certain types of content because platforms are private entities. Platforms, therefore, have the discretion to decide what legal content is permissible on their services. These challenges are unique to the U.S. legislative environment.
This brief outlines five categories of legislative approaches EU and U.S. policymakers have explored to regulate internet platforms’ algorithms, and makes recommendations on how legislative proposals can be improved. This brief provides examples of existing proposals to highlight the range of legislative approaches policymakers are considering, outline similarities and differences, and note areas of focus for potential transatlantic efforts to harmonize regulation. Policymakers on both sides of the pond (and beyond) should establish a baseline set of standards and regulations to provide users in both regions with similar rights and streamline oversight and compliance mechanisms.
Generally, EU and U.S. proposals for algorithmic regulation fall into five categories. Some legislative proposals include provisions from multiple categories.
- Expanding privacy controls and user protections from algorithmic systems
- Promoting transparency by encouraging disclosure of qualitative or quantitative data around the structure, use, and impacts of algorithmic systems
- Establishing requirements for internal or independent evaluation and oversight
- Modifying and/or repealing intermediary liability protections
- Prohibiting the use of harmful algorithms
In addition to novel approaches to promoting algorithmic accountability, lawmakers in both regions already have legal frameworks at their disposal. For example, U.S. lawmakers can—and should—enforce anti-discrimination statutes such as the Civil Rights Act of 1964 and the Fair Housing Act in the online environment. Advocates, including the National Fair Housing Alliance (NFHA) and government agencies, including the Department of Housing (HUD) have succeeded in holding platforms accountable for the impacts of their algorithmic systems using this approach.
Editorial disclosure: This brief discusses policies by Facebook and Google (including YouTube), both of which are funders of work at New America but did not contribute funds directly to the research or writing of this piece. View our full list of donors at www.newamerica.org/our-funding.
Expanding Privacy Controls and User Protections From Algorithmic Systems
Many of the algorithmic curation and personalization systems that internet platforms develop and deploy rely on the vast collection of user data. These companies use various mechanisms to collect granular personal and behavioral data. With this data, companies create detailed profiles of their users and serve them with “relevant” content, including advertisements. This enables companies to increase the time users spend on their services and subsequently deliver more advertisements to them. In this way, these data collection and profiling practices are vital components to platforms’ revenue generation, which is why platform business models are commonly referred to as the “surveillance capitalism'' business model. Several advocates have noted that platforms are often reticent to implement changes, such as stronger user privacy controls, because platforms have a strong financial incentive to collect as much user data as possible.
Internet platforms also use the data they collect to train and test their algorithmic models. Despite sustained civil society pressure, companies offer users very few controls to determine if and how their data is collected and used. When companies do offer controls, they are often difficult to access and navigate.
Given that user data is critical to developing and deploying algorithmic curation systems, policymakers have introduced legislation restricting how platforms can collect and process user data, and creating stronger privacy controls for users. Over the past several years, U.S. members of Congress have introduced almost a dozen varying privacy bills.
A handful of these proposed bills include language related to algorithmic systems. The Consumer Online Privacy Rights Act, for example, prohibits entities from engaging in deceptive or harmful data practices and from processing or transfering data on the basis of specified protected characteristics like race, religion, and gender. The bill also gives users the right to request the deletion, correction, and export of their data, and requires entities to provide users with a clear privacy policy and to obtain user consent before processing or transferring personal user data. These requirements are outlined in several other privacy bills. The Online Privacy Act similarly requires users to opt-in before a platform can process their personal data using a personalization algorithm, and would allow users to request human review of certain types of automated decisions which could pose significant privacy harms to an individual.
To fully address the myriad harms that algorithmic systems can cause, U.S. policymakers must start by passing comprehensive, federal privacy legislation. At a minimum, such legislation should encompass four elements: 1) strong, meaningful, and comprehensive privacy protections; 2) data practices that safeguard civil rights, prevent unlawful discrimination, and promote equal opportunity; 3) requirements for governments at all levels to protect and enforce privacy rights; and 4) opportunities for redress in instances of privacy violations. Federal privacy legislation could lay the groundwork for more thorough regulation focused on algorithmic systems going forward. In the absence of federal legislation, states such as California, Colorado, and Virginia have passed their own privacy laws. However, these laws do not provide sufficient privacy and civil rights protections. Therefore, a federal privacy standard is needed to provide comprehensive protections across industries and states.
In the EU, the General Data Protection Regulation (GDPR) governs data privacy and security, placing strict requirements on entities that process the personal data of or offer goods or services to EU citizens or residents, even if the entity is not located in the EU. The GDPR reflects many privacy best practices, including requiring entities to obtain “specific, informed, freely given, and unambiguous” consent from users before collecting and processing their data, limiting data processing to the purposes it communicated to users when collecting the data, and limiting collection and processing of data to data the entity requires for the process it is carrying out. The GDPR also imposes additional safeguards for the processing of sensitive categories of data, including health data and data related to race or sexual orientation.
According to the European Data Protection Board (EDPB), the GDPR covers any processing of personal data by an algorithm and creates several obligations for companies operating algorithmic systems. For example, Articles 13 and 14 establish specific notice requirements, which in the context of algorithmic systems would require entities to inform users that they are using an automated decision-making system, what the purpose of the system is, why and how it is processing their personal data, what the consequences of this data processing could be, and what their rights concerning their data are.
The GDPR also requires companies to be accountable to regulators and individuals, and to consider the likelihood that their algorithmic systems could generate significant harm. In some cases, companies must conduct Data Protection Impact Assessments (DPIAs), which evaluate whether the processing of personal data could result in a high risk to individuals’ rights and freedoms . Although the GDPR is not perfect—and there are ongoing debates around how certain provisions should be interpreted and enforced—it establishes a minimum standard for privacy protection in the EU, and both the DSA and AI Act proposals complement the GDPR. In this way, a foundation of privacy protections has enabled the European Commission to engage in more granular thinking around algorithmic regulation.
Promoting Transparency by Encouraging Disclosure of Qualitative or Quantitative Data Around the Structure, Use, and Impacts of Algorithmic Systems
Transparency has been a focal point of civil society and researcher advocacy with internet platforms. Advocates have particularly pushed companies to provide insight into the policies that guide platform use of algorithmic curation tools; how companies train, develop, and use these tools; and the impact of these tools on user experiences, rights, and online speech.
However, platform transparency efforts vary significantly in terms of their scope and granularity. In 2018, Facebook and Google (via YouTube) began publishing information about their content moderation policies and how their moderation efforts rely on automated tools. Some platforms also disclose limited information about how their ranking and recommendation algorithms work. However, questions remain, particularly around how platforms develop, train, and use these systems. Additionally, the information platforms share does not always paint a meaningful picture of their impact and how these systems operate. While platforms should disclose information relevant to their unique services, there is no standardization or consensus on transparency metrics, making comparison of how platforms use algorithmic systems for personalization and content curation purposes difficult.
EU and and U.S. policymakers have focused on promoting transparency around algorithmic curation systems through three main approaches:
- Increasing transparency around the use and operation of algorithmic systems: In the United States, the proposed Filter Bubble Transparency Act would require internet platforms using AI or ML-based systems to process user data for content personalization to notify users that they use their data to curate their experiences. The bill would also require platforms to allow users to opt-out of this algorithmically "filtered" version of the service. Similarly, the Algorithmic Justice and Online Platform Transparency Act would require platforms to explain to users what kinds of personal information they collect to enable algorithmic processes, how they collect this data, how they use this data to train or facilitate algorithmic processes, and how these algorithmic processes use this data to curate user's experiences.
As previously noted, the GDPR requires entities using an automated decision-making system to process personal data to notify users, explain how their data is used, and so on. The draft DSA builds on this in the content moderation context, requiring hosting services providers to give individuals who have had their content or accounts moderated—including by an algorithmic system—with adequate notice and access to an appeals process. The DSA also outlines that Very Large Online Platforms (VLOPS) using recommender systems must explain if and how users can adjust their recommendation settings, and offer at least one interface that does not use profiling. The draft AI Act similarly requires platforms using algorithmic systems that monitor and detect user emotions or other personal characteristics to inform users these systems are in use. Additionally, if an AI system is generating or manipulating content that appears authentic, the system provider must disclose to users that an algorithmic system artificially produced the content. Both of these provisions seek to tackle the potentially manipulative effects of algorithmic systems. However, the proposed AI Act does not require providers of high-risk AI systems to disclose information around how they train these systems—a significant transparency gap.
The GDPR also requires entities to provide privacy policies to users in clear and plain language. The DSA includes similar requirements for the policies that guide platforms' algorithmic curation systems. For example, intermediary services providers must outline their content moderation policies and explain how the platform uses algorithmic systems to moderate content. Additionally, platforms would need to share the primary parameters used to determine advertisement delivery. VLOPs must also share the primary parameters influencing their recommendation systems. Under the proposed AI Act, providers of high-risk AI systems must provide information about the system's performance characteristics, capabilities, limitations, and outputs to users. - Increasing transparency around the impact of certain algorithmic curation practices: Some U.S. legislative proposals aim to obtain greater transparency from platforms around how specific algorithmic curation practices shape user's experiences. The Algorithmic Justice and Online Platform Transparency Act, for example, requires platforms to issue transparency reports and an ad library to outline the scope and impact of algorithmic systems used for content moderation and advertising. Similarly, the Platform Accountability and Consumer Transparency Act requires platforms to issue transparency reports containing disaggregated figures on content it has algorithmically—or otherwise—moderated, demonetized, and downranked. These bills generally also solicit more insight into how platforms use automated tools to moderate content.
In the EU, the DSA includes similar stipulations, requiring intermediary services providers to publish, at minimum, one annual transparency report on its content moderation and curation efforts. Additionally, VLOPs that display advertisements must create an ad library with data on the nature, targeting criteria, and delivery details of advertisements run on their service. - Increasing researcher access to data: Both approaches discussed above focus on providing transparency around algorithmic systems to users and the public. This approach centers on providing vetted researchers with access to platform data, recognizing that this kind of expert analysis is critical for generating external accountability around platforms' algorithmic systems.
U.S. legislative proposals such as the Social Media DATA Act would require social media platforms of a specific size to create a library or database of all paid advertisements they run and provide academic researchers and the Federal Trade Commission (FTC) with access to the libraries. The bill also nominates the FTC to establish a stakeholder group to outline social media data sharing best practices with external researchers. Similarly, the Platform Accountability and Consumer Transparency Act tasks the National Institute for Standards and Technology (NIST) with constructing a voluntary framework that includes guidance on standards and processes for researchers seeking access to data on content that has been removed, demonetized, or deprioritized by a platform.
In the DSA, Article 31 outlines that researchers can submit requests for data related to illegal content, manipulative use of platforms, and certain categories of harms to the European Commission or the EU country hosting a platform. If a request is approved, VLOPs must comply. However, only researchers with academic institution affiliations or relevant expertise can access this data. The proposed AI Act does not include specific provisions related to data sharing with researchers. However, it notes that any prohibition on high-risk systems should not inhibit research on these systems for legitimate and ethical purposes. In the proposal, the European Commission also recognizes the importance of shared data spaces that enable the research and development of responsible high-risk AI systems.
Of all the approaches lawmakers have explored for promoting algorithmic accountability, the transparency interventions mentioned above are the most granular. This is because these proposals build on and reflect long-standing efforts from stakeholders such as civil society organizations to identify transparency metrics for algorithmic curation systems. However, for these legislative interventions to promote meaningful transparency that can translate into accountability, lawmakers must consider a few points.
Prioritizing quality over quantity: Transparency reports are a centerpiece of platform transparency advocacy efforts. However, platforms can not easily communicate all insights related to algorithmic systems through transparency reports alone, which rely on disaggregated quantitative statistics. Lawmakers must therefore pursue broader types of transparency from platforms, including qualitative transparency. Further, lawmakers must recognize that providing transparency, whether through a transparency report, ad library, or another interface, can be expensive, particularly for resource-strapped smaller platforms. Many existing transparency proposals include exceptions or different requirements for smaller platforms. However, lawmakers must collaborate with other stakeholders to refine these parameters and place reasonable requirements on smaller companies.
Accessible transparency: Currently, the handful of platforms that provide transparency around the design of their algorithmic curation systems and their algorithmic use policies often bury this information in blog posts and press releases, which are inaccessible unless a user knows what to search for, is willing to spend time looking for a specific output, and has an above-average understanding of algorithmic issues. Lawmakers should require platforms to communicate critical information in a more accessible and understandable manner.
Audience: Thus far, most legislative proposals aim to obtain transparency around algorithmic systems for a general audience. Going forward, legislative efforts should consider the target audience of these efforts and ensure disclosures are comprehensible by and appropriate for each audience. Additionally, as lawmakers work to establish data sharing and access regimes, they should reconsider how they define vetted researchers. As noted, the draft DSA excludes journalists and activists from its definition of researcher, although these stakeholders are critical in driving platform accountability.
Establishing Requirements for Internal or Independent Evaluation and Oversight
Internet platforms’ algorithmic systems operate as “black boxes.” This means both internal actors (e.g., product engineers) and external actors (e.g., advocates, policymakers, users) have a limited understanding of how these systems process inputs to generate outputs. This opaqueness has complicated efforts to obtain transparency, accountability, and oversight. To combat this problem, some advocates and researchers have championed the use of evaluations such as algorithmic audits and impact assessments. Depending on the design and implementation of these assessments, they can help promote greater fairness, accountability, and transparency around how platforms develop, train, and use black-box algorithms.
These accountability mechanisms have appeared in numerous U.S. legislative proposals. For example, the Algorithmic Accountability Act and the Mind Your Own Business Act specify that providers of high-risk automated decision systems must conduct an impact assessment to evaluate the system development process, including how the platform designed and trained the system; outline the costs and benefits of the system; and consider whether the system produces any adverse impacts related to accuracy, fairness, bias, discrimination, privacy, and security. Additionally, the Algorithmic Fairness Act would require entities to conduct and retain a five-year audit trail that includes information related to the data used by the algorithm, the methodology used to develop the algorithm, the data or datasets used to train the algorithm, and discriminatory effects across different subgroups. The Algorithmic Fairness Act focuses on algorithms that could impact opportunities to access critical areas of life, including education, housing, and credit. It also tasks the FTC with studying whether covered entities conduct audits and whether these auditing procedures and results are accessible to individuals or groups challenging determinations made by the entity’s algorithmic systems.
As previously noted, the GDPR requires certain providers, such as those deploying new technologies or technologies that have not been subject to robust assessments before, to conduct DPIAs. Entities must conduct DPIAs before processing data to understand the likelihood and severity of risk to users’ fundamental rights. The assessment must outline how the company will mitigate any identified risk and protect personal data in line with the GDPR.
In the DSA, VLOPs must conduct annual risk assessments evaluating systemic risks related to the operation of their services, including their content moderation, targeted advertising, and ranking and recommender algorithms. These assessments must evaluate three key elements: 1) the spread of illegal content on a platform; 2) whether the platforms’ operations cause any harmful effects related to fundamental rights such as freedom of expression and information; and 3) whether the platform has been intentionally manipulated through inauthentic behavior or automated exploitation in a manner that could cause adverse effects, including on public health and civic discourse. VLOPs must take reasonable steps to mitigate any risks identified in these assessments, and submit to independent audits to assess their compliance with the DSA.
In the AI Act, high-risk AI system providers must conduct conformity assessments—internal evaluations which ensure a provider is in line with the regulation. However, entities do not need to share the results of these assessments with the public.
These accountability mechanisms hold promise. But, for them to generate meaningful results, EU and U.S. lawmakers must address three issues:
- Lack of guidance and frameworks for evaluations: Although many legislative proposals require platforms to conduct algorithmic evaluations, these proposals provide little to no guidance on the structure of these evaluations, what the goals of these assessments are, and when during an algorithms’ lifecycle these evaluations should take place. Additionally, very few of these proposals require platforms to disclose the findings of these assessments to researchers or the public, thereby limiting their effectiveness. Some organizations—including Data & Society, the AI Now Institute, and the Ada Lovelace Institute—have proposed frameworks for such evaluations. For these assessments to generate meaningful outcomes, they must generate comparable results between similar platforms and be developed through multi-stakeholder dialogue. An entity such as NIST could lead such efforts. Clear standards are also important as they enable platforms to identify which data points they need to collect and ensure they can comply with any regulation. Such guidance will also help detail how various assessments, including audits, risk, impact, and conformity assessments, fit together and complement one another.
- No clear landscape for external evaluations: There is currently little guidance on who is eligible to conduct proposed algorithmic assessments. The DSA, for example, provides limited guidance—auditors must be independent, technically literate, and have relevant expertise in the area of risk management. As lawmakers seek to promote external evaluations of platform algorithms, they must consider which entities are best suited to perform such assessments and whether existing entities are well resourced and trained to perform such assessments at scale. The DSA notes that VLOPs must submit to compliance audits at their own expense. However, U.S and EU lawmakers have yet to contribute resources to develop and train reliable, independent entities who can perform such assessments. Lawmakers have also not yet supported creating appropriate standards of practice, credentialing mechanisms, and codes of conduct.
- Access to data: In situations where platforms must undergo independent, external evaluations, the lack of data-sharing structures can create complications. The DSA, for example, states platforms must give auditors access to all relevant data necessary to conduct the audit. It also requires auditors to maintain the confidentiality, security, and integrity of the data, including trade secrets. This is a good first step that should be emulated more broadly, including in the United States.
Modifying and/or Repealing Intermediary Liability Protections
Over the past several years, public controversies have revealed how internet platforms—and their algorithmic systems—can contribute to a range of harms, including disinformation, radicalization, discrimination, and election interference. As a result, many lawmakers have explored mechanisms for holding platforms liable for the outcomes of their algorithms. This legislative approach has mainly gained steam in the United States, with Section 230 of the Communications Decency Act as the focal point.
Section 230 provides intermediary liability protections to web hosts, social media networks, website operators, and other intermediaries who host user-generated content. The statute enables these providers to host and moderate content on their platforms without being held liable for the content of their users speech. As many free expression advocates have noted, these critical protections have allowed platforms, including today's largest social media companies, to thrive without fear of being held liable for the content of their users' posts. Some U.S. lawmakers have explored amending Section 230 to promote greater algorithmic accountability.
For example, the Civil Rights Modernization Act seeks to amend Section 230 to clarify that intermediaries do not have liability protection if they violate civil rights laws through their targeted advertising systems, which often use algorithms. Similarly, the Protecting Americans from Dangerous Algorithms Act amends Section 230 to hold platforms liable if their algorithms amplify or recommend content relevant to a civil rights case or acts of international terrorism. While certain civil society organizations have endorsed some efforts to amend Section 230, many have flagged that existing legislative proposals fail to adequately tackle algorithmic harms and promote meaningful accountability. Opponents of amending Section 230 note that these proposals mistakenly view the statute as a barrier to fostering algorithmic accountability and ignore the myriad existing proposals that could help promote transparency and accountability. Additionally, some advocates have noted that many of the current reform proposals fail to recognize the statute's critical role in promoting a free and open internet. Others warn these proposals may cause unintended consequences, including harming smaller platforms instead of holding big technology platforms accountable. Many civil society organizations are now exploring whether limited amendments of Section 230 could help augment algorithmic accountability without generating significant negative consequences. However, thus far, no Section 230 reform proposals have garnered widespread support. Going forward, lawmakers exploring this legislative approach must tailor proposals to adequately address algorithmic accountability issues without generating numerous negative externalities.
In the EU, intermediary liability interventions are not central to algorithmic accountability efforts. The E-Commerce Directive, adopted in 2000, establishes standards for intermediary liability protections across the EU. While the draft DSA includes some changes to that existing liability framework, it generally does not remove intermediary liability protections. It maintains, via the "Good Samaritan" principle, that intermediaries who voluntarily moderate potentially illegal user-generated content shall continue to receive these protections.
Prohibiting the Use of Harmful Algorithms
In some situations, EU and U.S. lawmakers have noted that certain algorithmic systems pose too much risk to society and should therefore be prohibited. In the United States, the Algorithmic Justice and Online Platform Transparency Act proposes prohibiting online platforms from using algorithmic processes to 1) segregate, discriminate, or make unavailable the “goods, services, facilities, privileges, advantages, or accommodations of any place of public accommodation;” 2) advertise opportunities that interfere with equal opportunity in housing, employment, credit, insurance, healthcare, and education; and 3) intentionally interferes with an individual’s voting rights, based on characteristics such as race and gender.
In the EU, the AI Act uses a risk assessment framework to segment high-risk AI systems into three categories: 1) systems that generate an unacceptable level of risk and should be prohibited, 2) systems that generate high-risk and should be subject to safeguards, and 3) systems that generate low or minimal risk and should be subject to less stringent safeguards. The draft regulation prohibits systems such as AI-based social scoring systems used by public authorities and “real-time” remote biometric identification systems used by law enforcement in public spaces, with some exceptions. The prohibition most relevant to internet platforms is related to systems that can manipulate individual behavior using “subliminal techniques” or exploit vulnerable groups—such as children or disabled individuals—in a manner that can cause them or another person psychological or physical harm. Some experts suggest this prohibition could include platforms’ ad targeting and delivery and recommender algorithms. However, the EU Commission needs to clarify this.
As lawmakers explore prohibiting certain types of algorithms, they should use a risk-based framework that enables them to adequately identify and articulate harms they wish to prevent or mitigate and which algorithmic systems correspondingly should be prohibited or subject to additional safeguards.
However, for such an approach to be viable, lawmakers must engage in multi-stakeholder dialogues to produce clear policy definitions for technical terms. In particular, there needs to be greater consensus around the definitions of terms such as algorithmic process, automated decision system, high-risk AI, and algorithmic use terms such as downranking. Vague definitions could result in the overbroad or narrow prohibition of algorithmic systems. Additionally, unclear definitions makes implementation of policy requirements challenging for technical teams.
Conclusion
Internet platforms develop and use algorithmic systems to curate and personalize online content. As the potential harms of these systems become more apparent and consequential, EU and U.S. policymakers have introduced numerous legislative proposals to promote greater transparency, accountability, and oversight over platforms and their systems. Thus far, the proposals seeking to expand privacy protections and user controls, promote transparency, and establish mechanisms for independent oversight are the most meaningful and viable, and policymakers should implement all three approaches together, while strengthening these measures per our recommendations. Should policymakers pursue legislation prohibiting certain algorithmic systems, they must provide clear processes for determining which algorithms should be banned. Further, lawmakers pursuing changes to intermediary liability structures must put additional thought into their legislative approaches, and ensure their proposals truly address the harms they are seeking to tackle.
Going forward, EU and U.S. policymakers should also collaborate to establish a common set of standards for algorithmic accountability for internet platforms, as this will confer a baseline of users' rights and help streamline compliance and oversight.