The Flaws in the Content Moderation System: The Middle East Case Study
Blog Post
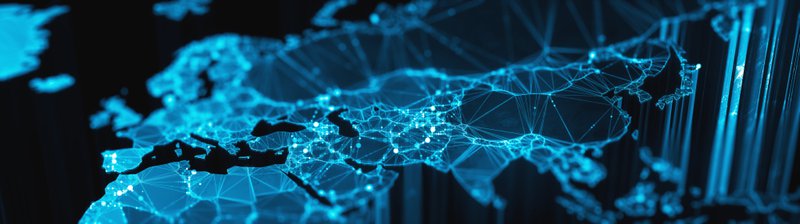
Shutterstock.com
Nov. 17, 2020
When major social media platforms were designed and launched over the past two decades, it is unlikely that those involved could have predicted the enormous power these tools would come to wield. It would have been difficult to imagine a world in which, for example, the well-being and survival of a gay man living in Egypt was tied up with an American social media company’s ability to flag, sensitively track, and respond to instances of violent hate speech on its platform. This, along with countless other examples, demonstrate the centrality of tech platforms like Facebook, Twitter, YouTube, and Google in questions of free speech, governance, and human rights in the Middle East. In particular, the question of content moderation — how platforms create and enforce policies which determine what kinds of user-generated content are and are not permissible on their services — has become a focal point of these discussions.
Technology companies have long been in the spotlight in the Middle East — both for good and for ill. These platforms have garnered attention for hosting and amplifying extremist content, playing a role in facilitating popular resistance movements before, during, and after the Arab Spring, and for serving as a lifeline for embattled journalists and activists for whom traditional media outlets are often inaccessible or censored. In many ways, the Middle East is a microcosm of how contemporary content moderation policies — and the social media platforms that create and implement them — can critically influence free speech and human rights and yield positive or catastrophically negative results.
Some new developments on long-standing political trends in the region make these content moderation issues all the more significant. The region holds some of the world’s worst records on questions of freedom of expression, and many regional governments are leaders in finding innovative ways to police speech and shut down opposition online. Turkey recently began imposing penalties under a new social media law that obligates platforms to, among other things, appoint a local content moderation representative with the power to block or remove content that the government deems unacceptable. This law has critical implications for the transforming relationship between technology platforms and the countries that use them, particularly in the case of non-democratic or partially democratic states. How platforms shape and implement their content moderation policies therefore has enormous consequences for the Middle East and its citizens.
In order to mitigate the spread of harmful content such as graphic violence and extremist content on their services, internet platforms have instituted massive content moderation programs that rely on both human moderators and automated tools. Human moderators — trained low-wage contract workers who work on massive teams and spend hours per day reviewing and flagging content that violates tech companies’ content policies — handle a large portion of this work. But the difficult and often traumatizing nature of the work, as well as the need to scale content moderation operations, have pushed companies to also develop and deploy artificial intelligence (AI) and machine-learning-based tools for content moderation.
Internet platforms often tout these algorithmic tools as silver-bullet solutions to content moderation issues, arguing that they can help companies scale and improve their content moderation operations with reliability and accuracy. However, this framing fails to consider the limitations of these automated tools. In particular, although automated tools are increasingly being deployed for content moderation and curation purposes, they are limited in their ability to make accurate judgments in situations where definitions around a category of content are vague and unclear. For example, when companies moderate child sexual abuse material (CSAM), which is globally regarded as illegal, they are more easily able to distinguish what kind of content does and does not fall into this category. As a result, it is far easier to train automated tools to accurately detect and remove this content. However, when it comes to moderating categories of content with more fluid delineations, such as extremist propaganda and hate speech, developing tools that can detect, let alone remove, this content with accuracy is extremely challenging.
In addition, these automated tools are further limited in their ability to detect and flag content that requires subjective or contextual judgment to understand. One of the most well-known cases of this is when YouTube began erroneously removing content posted by human rights and watchdog organizations seeking to document atrocities in Syria, mistaking the content for extremist propaganda. The removals were a result of Google’s adoption of new machine-learning technology designed to detect extremist content. However, the tools resulted in the mistaken removal of content shared by groups including the monitoring organization Airwars, open-source investigation site Bellingcat, online news outlet the Middle East Eye, and the Syrian Archive, an open-source platform that verifies and collects documentation of human rights violations in Syria. Despite these limitations, internet platforms do not provide adequate transparency and accountability around how they use these tools for content moderation and what the risks to user expression are. When platforms do share information, it is rarely region-specific, making it difficult for users in regions such as the Middle East to understand how exactly their speech is being impacted by these companies’ policies and practices. For this reason, it is vital that platforms provide impacted users with adequate notice and a timely appeals process that offers users a mechanism for remedy and redress if their content or accounts have been erroneously flagged or removed. Some platforms, such as Facebook, Twitter, and Google, offer these appeals processes. But more companies need to invest in providing this infrastructure going forward, in order to safeguard user rights around the world.
Companies also continuously fail to provide transparency around how the automated tools they use for content moderation are created, used, and refined, and how accurate they are. For example, in its latest Community Standards Enforcement Report (CSER), Facebook disclosed that its algorithmic tools are able to proactively detect 94.5% of hate speech before a user reports it to the platform. However, the platform has not published the success rate of individual language algorithms, making it difficult to discern whether the 94.5% figure is one that obscures lower success rates in certain languages. According to Facebook, the company operates hate speech classifiers in over 40 languages worldwide, including English and Arabic. However, these algorithms are trained using vast datasets, and robust data is often not available in less commonly spoken languages. As a result, harmful content that is spread in these languages, which often targets minority communities, must be manually flagged by users, who are often victims of the harmful speech itself. This creates disproportionate harms for already marginalized groups.
Further, although companies such as Facebook state that they do not have robust datasets for less-spoken languages with which they can train their algorithms, the company has shown that with enough commitment and drive, it can in fact improve its moderation technologies to better address the concerns of minority groups and languages. For example, following the Rohingya genocide and the spread of online hate speech targeting the Rohingya community in Burmese, Facebook invested significant resources to develop a hate-speech classifier in Burmese, hiring 100 Burmese-speaking content moderators who manually developed a dataset of Burmese hate speech that the company used to train its algorithm. However, without more granular data around how companies train their tools, and how accurate these tools are across different regional, cultural, and linguistic vectors, it is difficult to fully understand which communities and whose voices are being undermined during the content moderation process and where platforms need to invest more time and resources into improving these systems.
Because automated tools are limited in their ability to make accurate decisions in complex, contextual, and subjective situations, particularly in languages other than English, it is vital that platforms keep a human reviewer in the loop when moderating content. This is particularly significant in the case of the Middle East, where the predominant language is Arabic. Arabic is also part of the language of worship for the world’s 1.8 billion Muslims both in and outside of the Middle East, and therefore contains many complex use cases beyond everyday speech. It is also important to note that the Arabic language, a diglossic language that uses a standardized written dialect alongside a large variety of spoken colloquial dialects, presents another challenge for effective and fair content moderation. Although Facebook, for example, includes Modern Standard Arabic as one of the five languages used to train its content moderation tools, the complexity of diglossia in Arabic speech online is difficult to account for, and most likely is not captured or analyzed as accurately by AI models used to flag and remove content. This underscores the need for a human to be kept in the loop, particularly a native speaker with robust cultural knowledge and expertise that enables them to discern appropriately between different types of language use.
Unfortunately, accuracy and transparency concerns in content moderation across different regions also extend to human review. Today, platforms such as Google and Facebook have invested heavily in hiring and training thousands of outsourced, offshore, and in-house content moderators. However, once again there is a fundamental lack of transparency around how these moderators are trained, and in which regions, cultures, and languages they are competent. There is also little public data around how quickly content flagged in different languages is addressed. As mistaken removals of content and accounts belonging to activists, journalists, and average users continue to be documented on platforms such as Facebook and Twitter, it is evident that companies must invest more in ensuring that users from regions such as the Middle East do not have their free expression rights routinely and erroneously undermined because of gaps in platform policies and procedures.
The Middle East’s linguistic complexities, as well as instances of increased digital surveillance and crackdowns on freedom of information, suggest that many countries in the region are at an inflection point with regard to content moderation and the limits of speech online more broadly. As outlined, the content moderation policies and practices that major U.S. internet platforms create and deploy can have significant consequences in the Middle East. As a result, a regional studies approach to content moderation and governance, which accounts for local cultural and linguistic sensitivities, is crucial to holding tech giants accountable, particularly as their roles of gatekeepers of online speech expand. The creation and identification of lexicons of region-specific hate speech in lesser-used languages, for example, is one step toward the type of work that would make this regionally-rooted and locally-based approach possible. The Middle East can, in this sense, serve as a crucial test case for how a regionally-rooted approach to content moderation on the world’s social media platforms will be central to those companies’ larger public policies, and have significant consequences for the future of free speech, journalism, and beyond.
In this series, published jointly with the Middle East Institute, we will examine how content moderation and social media policies and practices intersect with regional issues in the Middle East, and how these linkages can influence security, civil liberties, and human rights across the region and beyond.